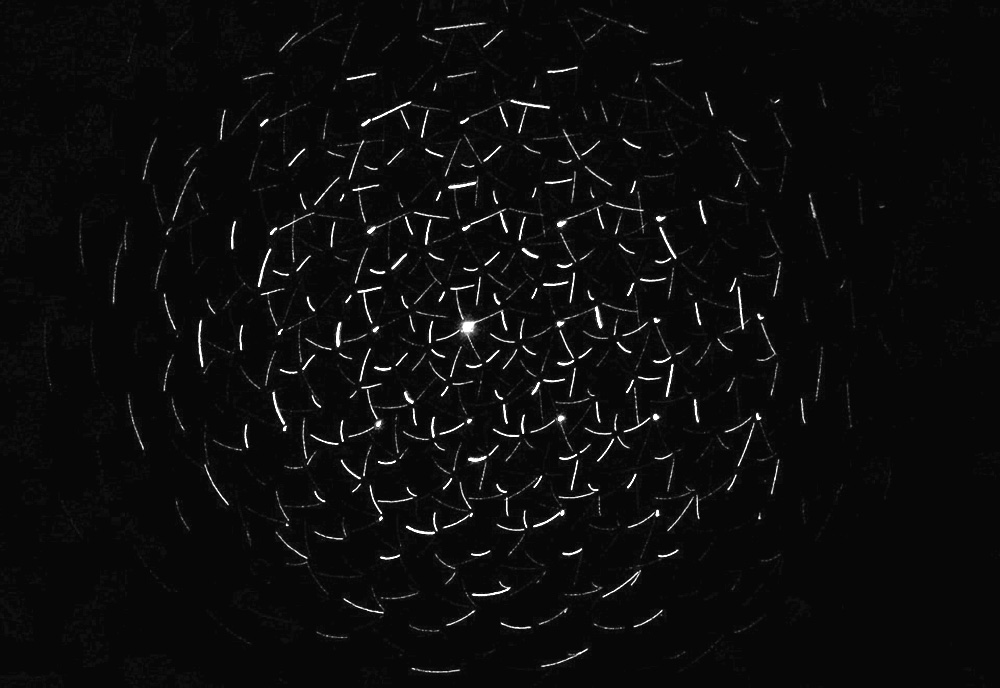
Correlation and college tumors
Most of us are familiar with the adage that “correlation does not imply causation.” So it is mildly maddening to see a newspaper run the headline: “Why Going to University Increases Risk of Getting a Brain Tumor.” No, the study did not find a direct link between exam-cram sessions and abnormal growths inside our skulls. As the statistician David Spiegelhalter explains, the news article had simply neglected the fact that “wealthy people with higher education are more likely to be diagnosed and get their tumor registered.”
In his book, The Art of Statistics, Spiegelhalter laments our tendency to ignore “confounder effects” in our interpretation of relations and statistics. There is, for example, a correlation between the sales of ice cream and the number of drownings. But you would not conclude that ice cream must therefore be the culprit. We would look instead to the influence of weather on human behavior. While that’s an obvious example, confounders lurk everywhere. So we have to be vigilant.
Framing and priming
It does not help either that we are malleable to framing and priming. As Spiegelhalter recounts, when a pre-Brexit survey “asked people whether they supported or opposed ‘giving 16- and 17-year-olds the right to vote’ in the referendum on whether to leave the European Union, 52% supported the idea.” But “when the same respondents were asked the (logically identical) question of whether they supported or opposed ‘reducing the voting age from 18 to 16’ for the referendum, the proportion supporting the proposal dropped to 37%.” Now imagine the seriousness when every question or statistic we encounter in life is framed and primed in some way. Our interpretation is often shaped in ways that we do not realize.
Unavoidable variability
Indeed, we have to remember that “unavoidable variability underlies everything interesting in real life”, writes Spiegelhalter. We know today, for instance, that smoking cigarettes increases the risk of lung cancer. But it took some time for the medical establishment to accumulate evidence and reach consensus. Some smokers get lung cancer while others do not. “So our ‘statistical’ idea of causation is not strictly deterministic… Signals always come with noise.” (And this is true even before we get to the vested interests of corporations, advertising agencies, and lobby groups that seek to impose their own vision on empirical truth.)
Moreover, variability means that runs and streaks can arise by pure chance. So when the government installs new speed cameras after a recent spate of crashes, and celebrates the subsequent fall in accident rates, one must wonder if it was sheer luck. Similarly, when the head coach is replaced after a series of disappointing losses, we should not be quick to credit his or her successor with the team’s resurgence. Sometimes, the process is simply in line with what the statistician Francis Galton would call “regression to the mediocrity” (i.e., regression to the mean).
Spurious models and overstuffing
Today, we have an abundance of models. Random forests, support vector machines, neural networks, and related machine-learning algorithms help us to traverse the vast oceans of data and uncertainty. But complex models come with their own risks. For one, we must not forget that algorithms rely on statistical associations. They can be sensitive to spurious relations and implicit bias. Spiegelhalter points to one example in which a vision algorithm was trained to distinguish between photos of huskies and German shepherds. The algorithm appeared to run well until it failed to see the huskies that owners kept indoors. The programmers found to their surprise that the algorithm had simply learned to spot snow instead of dogs. While this was an innocuous case, the dangers of algorithms are manifold if they are applied inappropriately to public policy, the courts, and so on.
Now, it is tempting to address such issues by adding more and more details to our models to explain every nook and variation. But as the Linda problem in probability theory illustrates, additional details may not confer explanatory power. We might actually be trading reasonable accuracy for a false sense of precision that appeals to our storytelling instincts. Spiegelhalter adds that “by making an algorithm too complex, we start fitting the [model to] noise rather than the signal.” Not only that, complex models tend to grow opaque. They become hard to trace, fine-tune, upgrade, and communicate. “All this points to the possibility that quantitative performance may not be the sole criterion for an algorithm.”
Type I and Type II errors
Similarly, much emphasis is placed today on statistical significance. Some researchers and students, for example, will often hunt for low P-values. (The measure tells us “the probability of getting a result as extreme as we did, if the null hypothesis were really true.” So “if a P-value is small enough, then we say the results are statistically significant.”) Spiegelhalter reminds, however, that this is conditional also on the assumptions that underpins the statistical model. For linear regressions, they include linearity, homoscedasticity, independence, and normality. Statistical significance may suggest that we’ve found something, or that our specification was wrong.
Moreover, when it comes to hypothesis testing, we have to remember that two types of errors are possible. A Type I error is a false-positive. This is where the null hypothesis is true, but we reject it in favor of the alternative. A Type II error is a false-negative. This occurs when we fail to reject the null hypothesis when the alternative hypothesis is true. Spiegelhalter likens these errors to a courtroom analogy—where “a Type I legal error is to falsely convict an innocent person, and a Type II error is to find someone ‘not guilty’ when in fact they did commit the crime.”
To borrow his example, imagine that only 10 percent of the null hypotheses that we investigate are false; and that the probability of a false-positive and false-negative is 5 percent and 20 percent respectively. This means that for every 1,000 studies conducted (Figure I), we’d expect to make 80 correct ‘discoveries’, claim 45 discoveries incorrectly (Type I error), and miss 20 ‘discoveries’ (Type II error). Variability and error adds noise to signal.
Figure 1: Error rates and hypothesis testing
State / Results | Reject null hypthesis | Do not reject null hypothesis | Total studies |
Null is false | 80 (‘Discovery’) | 20 (Type II error) | 100 |
Null is true | 45 (Type I error) | 855 | 900 |
Total studies | 125 | 875 | 1,000 |
Delusions of discovery
The Reproducibility Project, for example, replicated a hundred studies in psychology with larger sample sizes. As Spiegelhalter notes, “the project revealed that whereas 97 percent of the original studies had statistically significant results, only 36 percent of the replications did.” What’s more, “23 percent of [the] original and replication studies had results that were significantly different from each other.” The disparity in results highlights the importance of replication and reproducibility.
It serves also as a reminder of the pressures that academics face and the bias it creates for positive results. Indeed, many studies are rejected because they conflict with the organization’s objectives, or are not deemed to be compelling enough for publication. We should remember, however, that “statistical significance does not measure the size of an effect or the importance of a result.” As Spiegelhalter notes, there can be a difference between statistical and practical importance. “Obsessive searching for statistical significance can easily lead to delusions of discovery.” Small samples, leading questions, systematic biases, unaccounted confounders, incorrect models, selective reporting, and plain-old mistakes may add only to these dangers.
We should add, as an aside, that Type I and II errors apply not only to hypothesis testing and legal errors. False-positives and false-negatives arise everywhere, from medical diagnostics to classroom examinations to hiring decisions. In statistics, techniques like the Bonferroni correction and independent replication of studies may help to minimize false-positives. This is why we run multiple diagnostics, examinations, clinical trials, and job interviews. Unfortunately, in some high-stake situations like nuclear warfare, counter-terrorism and armed-conflict, those in charge may not have the time, resources, or clarity to discriminate between positives and false positives. The missiles may fly automatically, as they nearly did during the Cold War.
Precarious probabilities
It goes without saying that dealing with variability and uncertainty is a precarious affair. Spiegelhalter humbly admits that despite his decades of training as a statistician, he too needs time, pen and paper to handle basic questions in high school probability.
While transparency, reproducibility, and peer-review are bulwarks against bad statistics, we need to train our noses for misapplication. Spiegehalter himself proffers several routine questions: “How rigorously has the study been done?” “What is the statistical uncertainty in the findings?” “How reliable is the source?” “Is the story being spun?” “What am I not being told?” “What’s the claimed explanation for whatever has been seen?” “How does the claim fit with what else is known?” “Is the claimed effect important?”
In the end, everything we ponder about is laced with assumptions. This is true even for simple coin-flips. We naturally assume that every other potentiality, from the chances of an unexpected earthquake to the sun imploding, are not material enough to influence the outcomes of heads or tails. Indeed, a good model is like a good map. They must be simple yet rigorous enough for the territory ahead. As the statistician George Box would say, “all models are wrong, but some are useful.”
Sources and further reading
- Spiegelhalter, David. (2019). The Art of Statistics: How to Learn from Data.
- Newman, Mark. (2004). Power Laws, Pareto Distributions and Zipf’s Law.
- Mandelbrot, Benoit., & Hudson, Richard. (2004). The Misbehavior of Markets.
- Mlodinow, Leonard. (2008). The Drunkard’s Walk.
- Firth, William J (1991). Chaos—Predicting the Unpredictable.
- Stewart, Ian. (2019). Do Dice Play God?
Latest post
- The Trusted Advisor — David Maister on Credibility and Self-Orientation
- How We Learn — Stanislas Dehaene on Education and the Brain
- Catching the Big Fish — David Lynch on Creativity and Cinema
- Donald Murray on the Apprentice Mindset and Return to Discovery
- The Hidden Half — Michael Blastland on the Unexpected Anomalies of Life
- Artificial Intelligence — Melanie Mitchell on Thinking Machines and Flexible Humans
- Fallibility and Organization — J. Stiglitz and R. Sah on Hierarchies and Polyarchies
- The Art of Statistics — David Spiegelhalter on Reasoning with Data and Models
- Chess Vision and Decision-Making — Lessons from Jonathan Rowson
- The Drunkard’s Walk — Leonard Mlodinow on Randomness and Reasoning