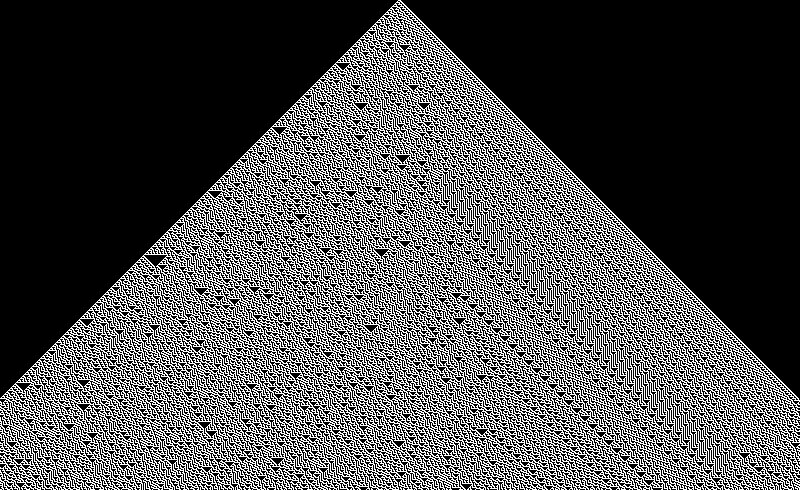
Reduction and construction
Science is a lot like mapmaking. We are trying to distil a large, messy picture into something manageable. The mapmaker’s challenge is in knowing what to leave out. But there’s a little more to it. A focus on reducible components may not give us a complete understanding of the whole. Simple micro interactions, as we know, can lead to complex macro phenomena.
As John Miller observes in A Crude Look at the Whole, if we seek to understand complex systems in economics, biology, ecology, and so on, reductionism is not enough. We have to incorporate concepts like construction, feedback, and emergence into our mental models.
“We inhabit a world where even the simplest parts can interact in complex ways, and in so doing create an emerging whole that exhibits behavior seemingly disconnected from its humble origins. … Reduction gives us little insight into construction. And it is in construction that complexity abounds.”
John Miller. (2015). A Crude Look at the Whole.
Automata, sea snails and invisible hands
Miller himself begins with the hypothesis that “more is different”: that new phenomena can emerge from the aggregation of simple, local interactions. Von Neumann’s cellular automata (Figure 1) and Conway’s game of life show, for example, how simple mathematical rules and interactions can produce complex structures, patterns, and dynamics.
Figure 1. Cellular automata (rule 30)
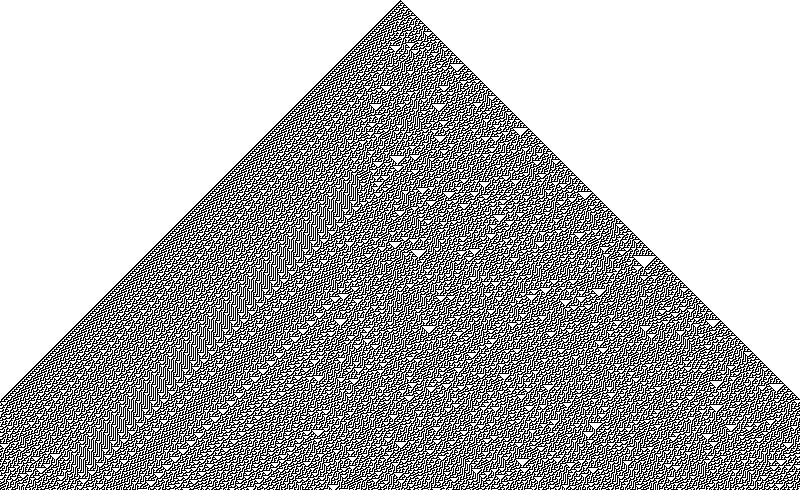
You can find many instances of local rules and global patterns in nature and society. Miller points, for example, to the dazzling patterns we see on sea snail shells. Their intricate designs are not a product of some master plan, but of “localized chemical interactions [that] determine the pigmentation of added cells”.
Likewise, in economic systems, there is the invisible hand of the market — aggregating and coordinating the interactions of decentralized buyers and sell. Prices adjust to all this hubbub, which in turn helps with the allocation of scarce resources, or so the theory goes. And for the most part, it seems to work reasonably well.
Stabilizers, amplifiers, and feedback loops
Miller writes also about the role of feedback effects in the dynamics and evolution of complex systems. You can imagine, for example, how mutational improvements in the camouflage of moths might affect its fitness, the fitness of its predators, and their evolutionary histories in turn. (Advances in human stealth and detection technologies exhibit similar patterns too.)
Similarly, as George Akerlof and Robert Shiller write in Animal Spirits, we want to distinguish between negative (self-regulating) and positive (amplifying) feedback. In commodity markets, for instance, the price system tends to exhibit its own stabilizer. Given a fixed supply, a rise in demand is usually met with higher prices (dampening part of the rise in demand).
Markets may sometimes exhibit positive feedback loops as well. In stock markets, a rise in price for some asset class may raise investor’s enthusiasm and expectations. This can lead to even greater demand, higher prices, and market enthusiasm. Other amplifiers, like deregulation, leverage, and the fear of missing out, may add further to the feedback loop.
Unintended consequences, Miller notes, are features of interactions and feedback. Additions and alterations to complex systems introduce all sorts of unforeseen complexities. Traders, for example, may recall the 2010 Flash Crash, in which new high-frequency algorithmic trading led to a hot-potato volume effect that plunged markets into a momentary tailspin.
“Sometimes feedback stabilizes the system, as happens when we install a not-too-touchy thermostat to control the furnace. Other times feedback causes a system to go out of control, as happens when we place a microphone too close to a loudspeaker, producing an ever-increasing screech.”
John Miller. (2015). A Crude Look at the Whole.
Heterogeneity and polycultures
The degree of heterogeneity among agents may also affect the nature of interactions and feedback in a system. Imagine, for example, what might happen to market prices, or voting in a political race, when the participants are homogenous. Because identical people will behave the same, collective behaviors might shift drastically when the trigger is sufficiently large.
A system of heterogeneous agents, by contrast, may experience a more graduated response (as their triggers are activated at different moments). That’s not to say that heterogeneity leads to steady or piecemeal dynamics. It depends a lot on the distribution of agents and the nature of their response rules.
A small protest, for example, may lead to revolution in a heterogenous population if the original group inspires more and more people to join the cause. A homogenous group, by contrast, may galvanize quickly or not at all depending on their sensitivity to the original event. You might liken this to the spread of infectious diseases in monoculture and polyculture crops.
Brains and thinking everywhere
Further down the rabbit hole, Miller notes that “we likely are surrounded by brains everywhere”, just not in the anthropocentric sense of the word. Indeed, many systems in nature have developed ingenious mechanisms for decision-making. Miller describes, for example, how single-celled organisms use simple response rules to chemical stimuli to navigate their environments (also see cell learning mechanisms).
“If signals and reactions are all that are needed for thinking, then brains may not be restricted to individuals. Perhaps larger-scale social systems, such as honeybees in a hive, people in an organization, or a collection of interconnected markets, may be performing thinking-like computations.”
John Miller. (2015). A Crude Look at the Whole.
Spectacular examples, I think, are found in social insects. Bees, for instance, utilize broad search, communication tactics (waggle dances) and feedback loops to converge on rich food supplies or to find a new home. Abstract away from the individual bees and their decentralized network begins to look a lot like an alien brain — an emergent superstructure born from simple interactions, response rules, and emergent behaviour.
“A honeybee worker has a limited set of behaviors tied to cleaning the hive, nursing brood, forming comb, collecting nectar and pollen, and so on. However, place thousands of such workers into a colony, and we get an entirely new set of useful, colony-level behaviors. Out of the interactions of thousands of honeybees emerges a superorganism capable of a survival-tested behavioral repertoire.”
John Miller. (2015). A Crude Look at the Whole.
Tangled hierarchies and emergent superstructures
Indeed, emergent structures appear to be a recurring feature of complex systems. From genes, species, and ecosystems, to people, companies, and economies, self-organization emerges on multiple levels, interlocked in a tangled hierarchy we do not fully understand.*
This applies also to the emergence and evolution of cooperation in nature and society. Single shot games like the prisoner’s dilemma or the tragedy of the commons can make cooperation look improbable. We know, of course, that this isn’t the case.
In Beyond States and Markets, Elinor Ostrom describes how decentralized rural communities overcome their common resource dilemmas through a web of communication, trust, monitoring, and graduated sanctions.
Miller similarly describes how “elaborate religious institutions” and annual congregations at their water temples helped downstream and upstream farmers in Bali to cooperate on the preservation of scarce resources.
Similarly, in Sapiens, Yuval Harari describes humankind’s propensity to invent superstructures to solve our affairs. History provides a good record of the many political, religious and monetary institutions we’ve tested. Capitalism and democracy too might be intermediate points in the cultural evolution of our social structures.
It goes without saying that cooperation emerges and persists because it is relatively advantageous to do so. Whether in nature or society, there is an incentive to cooperate when the shadow of the future from doing so is sufficiently large.
*The term “tangled hierarchy” comes from Douglas Hofstadter’s wonderful book I Am A Strange Loop.
The cosmic algorithms of life
These organizing principles and feedback loops hint at deeper rules that underlie the general features of complex systems. “Adaptive agents”, Miller suggests, “are part of a cosmic algorithmic dance, subject to deep forces that determine their fate”.
What we are trying to find are algorithms to complex problems. Miller likens it to invention of a novel drug, tasty beverage, or fashionable outfit. There are just so many combinatorial possibilities and nonlinear interactions to consider.
Miller describes, for example, how simulated annealing algorithms, which combine iterative search and error tolerance, can be used to find global optimums to complex problems.^ It’s like climbing a rugged mountain in thick fog. We want to reach the apex. But it isn’t obvious to us if we’re on the peak already or some local hill.
As Miller explains:
“Complex systems involve a very different kind of search, where the mountain range is not only rugged but also fog-bound, and perhaps even undulating with every step we take… In such a world, even if we hike up the fog-bound hill without error, we might miss the highest point on the landscape. Indeed, we might find ourselves victoriously standing on top of a molehill, falsely thinking that we have made it to the top of the mountain. To avoid making mountains out of molehills, we need new ways to search in complex worlds. In particular, introducing errors into our search process—that is, occasionally taking random steps downhill—may allow us to escape the trap of the molehill and head to the mountaintop.”
John Miller. (2015). A Crude Look at the Whole.
A grandeur view
Perhaps the grandest of these algorithms, outside that of physical law, is “Darwin’s keen insight” — how reproduction, variable inheritance, and natural selection has taken life from its simple, humble origins into bewildering complexity and biodiversity.
But if we’re to make further headway in our understanding of brains, superorganisms, economies, and whatnot, we are going to need a healthy mix of reduction and construction. As Miller notes, we do ourselves a disservice if we leave complexity and nonlinearity in a quiet corner of the sciences.
“There is grandeur in this view of life, with its several powers, having been originally breathed into a few forms or into one; and that, whilst this planet has gone cycling on according to the fixed law of gravity, from so simple a beginning endless forms most beautiful and most wonderful have been, and are being, evolved.”
Charles Darwin. (1859). The Origin of Species.
^ Miller also suggests that “the mechanisms that drive adaptive systems… have direct analogs to the key elements of the MCMC (Markov chain Monte Carlo) algorithm”. MCMC is a little more technical and involved, which we leave to you for further reading.
Sources and further reading
- Miller, John. (2015). A Crude Look at the Whole.
- Akerlof, George., and Shiller, Robert. (2009). Animal Spirits.
- Ostrom, Elinor. (2009). Beyond Markets and States.
- Robert Axelrod. (1984). The Evolution of Cooperation.
- Darwin, Charles. (1859). The Origin of Species.
- Hofstadter, Douglas. (2007). I am a Strange Loop.
Recent posts
- What’s Eating the Universe? Paul Davies on Cosmic Eggs and Blundering Atoms
- The Dragons of Eden — Carl Sagan on Limbic Doctrines and Our Bargain with Nature
- The Unexpected Universe — Loren Eiseley on Star Throwers and Incidental Triumphs
- Bridges to Infinity — Michael Guillen on the Boundlessness of Life and Discovery
- Ways of Being — James Bridle on Looking Beyond Human Intelligence
- Predicting the Unpredictable — W J Firth on Chaos and Coexistence
- Order Out of Chaos — Prigogine and Stenger on Our Dialogue With Complexity
- How Brains Think — William Calvin on Intelligence and Darwinian Machines