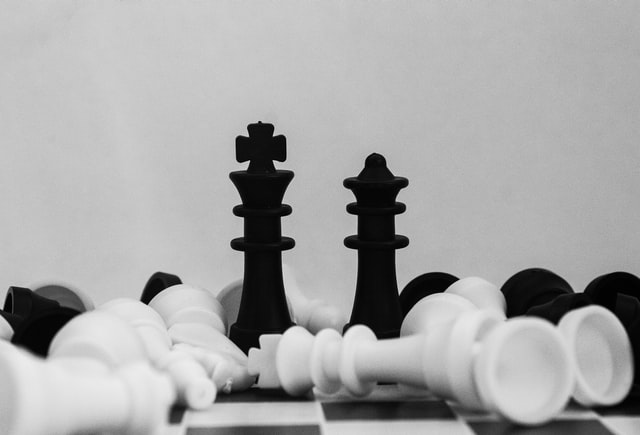
Lessons from the Grandmaster
Chess, in my opinion, is a fantastic microcosm for decision-making. Amongst many things, it provides a helpful mental model for competition, strategy, advantage and positioning. One of my favourite books in this domain is Deep Thinking by Garry Kasparov, in which the former chess Grandmaster recounts his matches with IBM’s supercomputer Deep Blue.
Deep Thinking rightly deserves its name. Kasparov has many insightful things to say, not only in chess, but on mastery, strategy, business, intelligence, technology, and so on. This post will cover the big lessons I took from Mr. Kasparov, from his approach to sustained excellence, to his views on the “gravity of past successes”. And as you can tell from the length of this post, I learned a great deal from him.
Skip ahead
- Kasparovian approach
- The Magician from Riga
- Skill and margins
- The chess machines
- Problems in search
- Alien Grandmasters
- Gravity of past success
- Human versus machine
- The main line
Kasparovian approach
Kasparov doesn’t have any general tips or tricks for success or disciplined thinking. Since everyone is different, what worked for him may not work for others. That said, there are three traits about Kasparov that really stand out to me: (1) his desire for new challenges; (2) disdain for losing; and (3) superior work ethic and discipline.
New challenges
Kasparov believes that the desire to seek uncomfortable challenges and new methods distinguishes the great from the good. Great athletes, executives, and companies focus not only on improving their strengths, but on their weaknesses as well. This requires some amount of risk seeking. While maintaining the status quo is comfortable and tempting, it often leads to stagnation over time.
“[When asked] what I thought separated me, the world champion, from other strong chess players, I answered, “The willingness to take on new challenges,” the same answer I would give today.”
Garry Kasparov, Deep Thinking, 2017
A sore loser
Kasparov’s disdain for losing was another driving force. While the joys of passion and victory are powerful motivators, “to be the best in any competitive endeavour you have to hate losing”. Kasparov admits that he is a sore loser. He hates losing in all forms, whether it’s against world champions or bad players, in good games or bad games, in chess or trivia.
One’s mental game, especially in the face of losses and setback, is critical to success. Players that find themselves unable to overcome the emotions of tough losses will cede momentum and confidence to their competitors. The onset of depression, excessive pessimism and the lack of self-confidence can inhibit one’s capacity to make decisions effectively.
“A match is not a test of one’s absolute ability to play chess… but of how well one has played those particular games. Therefore, the ability to monitor and control one’s mood is of great importance in determining the match outcome… [This] sort of mental robustness is rare, even among elite Grandmasters.”
Garry Kasparov, Deep Thinking, 2017
Botvinnik’s work ethic
Kasparov says there’s no secret to his achievements, “only innate gifts, hard work, and discipline”. The latter of which he credits to his mother, and to his teacher Mikhail Botvinnik (another former Grandmaster). He describes how Botvinnik would spend “time doing almost nothing but analysing the matches he lost… with an intense regime of self-criticism”. As Botvinnik himself wrote: “Conceit does not put one in the right frame of mind for work.”
Hardwork is a talent
To reach the level of Grandmaster in chess requires years of empirical study, knowledge, and experience. There are thousands of openings, endgames, tactics and positional patterns to understand. In this context, hard work in Kasparov’s view is a talent. And like any other talent, the talent of hard work requires cultivation.
Preparation is key to any Grandmaster’s success. Kasparov would scrutinise the tendencies, strengths, and weaknesses of his opponents. He would also evaluate how his own tendencies and biases influenced his analysis of games. Kasparov says that the temptation to repeat an old strategy without any improvement or innovation is equivalent to preparation for a loss. Like many other fields in life, chess is a game of skill and adaptation.
Here’s an excerpt from Kasparov that I really liked:
“Even when I was very young, I enjoyed studying the openings and searching for improvements to add to my arsenal. The exciting cut-and-thrust tactics of the middlegame get most of the attention, but the tenacity and ingenuity required to find a new idea in the well-trodden paths of the opening always attracted me. I studied my opponents’ openings comprehensively in search of weaknesses and kept huge database files full of novelties and analysis… I accept reserving the highest praise for over-the-board brilliance, but there is nothing to be ashamed about out preparing your opponent.”
Garry Kasparov, 2017, Deep Thinking.
The Magician from Riga
I found Kasparov’s account of a professional’s approach to chess fascinating. For instance, the opening phase of chess comprises largely of study and recall. Grandmasters select openings from their “personal mental library”, subject to their preferences and preparation. By contrast, move generation, at least to Kasparov, is more visuospatial than mathematical. He visualises the moves and positions first, and calculates after for verification and evaluation.
Tal’s intuition
Kasparov recalls a particular game of Mikhail Tal (nicknamed “The Magician from Riga”), in which the newspaper described Tal’s path to victory: “after carefully thinking over the position for 40 minutes, [Tal] made an accurately calculated [knight] piece sacrifice”. The reality, as Tal recounts it, was that he could not calculate all the possible variations. He made the knight sacrifice on pure intuition, and “could not refrain from making it” because “it promised an interesting game”.
Kasparov suspects that great chess players “rely on such intuitive leaps more often than [they] would like to admit”. While he believes in the power of human intuition, books like Daniel Kahneman’s Thinking, Fast and Slow, and Dan Ariely’s Predictably Irrational have “shaken” his belief in it. As Kasparov puts it: “after reading their work, you might wonder how we survive at all”.
But I believe that Kasparov’s definition of intuition, at least in chess, is very different from the layperson’s use of it. For Kasparov’s intuition is the product of decades of experience, learning and relearning. It’s not an uninformed gut feel. As Jared Tendler wrote in The Mental Game of Poker, the distinction between unconscious competence and incompetence is important. The former is cultivated, while the latter is not.
That said, Kasparov rightly points out how intuition can sometimes fail us. He gives the example of the Monte Carlo Fallacy. When a fair coin flips heads twenty times in a row, some will believe that the trend of heads is likely to continue. Others might believe that tail flips are now more likely, expecting some regression to the mean. In this case, each coin flip is independent of one another. The odds of heads or tails on the next flip is always 50:50. Some awareness of common fallacies and cognitive blind spots can help us to avoid them.
Skill and margins
In a game of skill, where the margin for error is slim, every minor advantage counts. Kasparov notes that even the most legendary of chess matches between Grandmasters are “riddled with mistakes and inaccuracies”. He believes in the old adage that victory goes to those who make the least errors. Here, there are three interesting ideas from the author that I’d like to note: (1) Lasker’s edge, (2) first mover advantage and (3) twin creations.
Lasker’s edge
Kasparov cites the work of Emanuel Lasker, who pioneered the role of psychology in chess strategy. That is, to play the moves that make your opponent uncomfortable. Understanding the technical and psychological tendencies of your opponent soon became popular in chess.
Over generations, some players began to use psychology to deceive or fluster their opponents. A quick move could signal confidence, while a long but confident pause may convey a new inspiration. Programmers even designed their chess machines to select between instantaneous and delayed reactions for psychological impact.
Kasparov believes that defending against mind games requires preparation, confidence in your preparation, and having your competitors know that too. He cites former Grandmaster Bobby Fischer, who said, “I don’t believe in psychology. I believe in good moves.”
First mover advantage
Similarly, Kasparov highlights the value of the first mover advantage. For amateurs, playing white (first) or black (second) confers little to no advantage. But for Grandmasters, that sliver of tempo from going first matters, since victory tends to go to the player who lands the first successful attack. While around half of all chess games are drawn at the professional level, the white pieces wins around twice as much as the black pieces. Again, where the margin for error is small, and the skill of competition elite, the value of minor advantages increases.
Twin creations
Kasparov tells readers that “at every moment of a chess game, the position is a joint creation”. At the highest of levels, “there is the assumption that he sees roughly what I see and is unlikely to be sure of anything I am not sure of myself”. During an opening game, both players might find themselves content with their development and positioning. One should be curious as to why either player’s confidence is justified.
The chess machines
Today, it’s difficult to distinguish between human Grandmasters and the elite chess programs. However, unlike human play, elite machines appear to fluctuate between superior play and “grotesque blunders” — a consequence of their design and algorithms. So, it’s interesting to compare and contrast chess programs with that of human Grandmasters. Both have achieved mastery and excellence through very different journeys and processes.
Kasparov says the formula for chess playing machines hasn’t changed too much since its early incarnation decades ago. While the problems, operations and goals of chess are well-defined, the solution is far from straightforward. It’s a wonderful testing ground and starting point for machine intelligence.
Minimax
Every chess program requires a set of guiding policies, evaluation functions and search mechanisms. Early developments in chess search theory focused on minimax algorithms. Minimax systems evaluate the possibilities and rank them from best to worst. Its evaluation function attaches a value to every variation it considers, while its rules and guiding policies help to determine how it thinks under different permutation and environments.
Type A and Type B
There are two primary types of search techniques for move selection: brute force (Type A) and intelligent search (Type B). Type A is exhaustive, seeking to evaluate every possible permutation and possibility that it can. By contrast, Type B relies on guiding policies and an efficient algorithm to scrutinise and select from a smaller pool of possibilities.
Human decision-making shares similarities with Type B search. We rely on experience, intuition, and pattern recognition to identify the set of possibilities in which to focus. We lack the memory bank and computational power to brute force our way through problems. Chess, like other real-world problems, is far too complex to explore every permutation.
Here’s a snippet into Kasparov’s mind when playing chess:
“When I look at a chess position, … there is very little that is consciously systematic about my move search process… Disregarding forced moves, each position will have three or four plausible moves, sometimes as many as ten or so. Again, before any real search begins in my mind, I have selected several to analyze more deeply, what we call candidate moves. Of course, I’m not starting from scratch if it’s my own game; I’ve been planning my strategy and looking at the most likely variations… And often I will plan out a sequence of four or five moves in advance, only pausing to double-check my calculations if the sequence plays out as expected.”
Garry Kasparov, 2017, Deep Thinking.
Null moves
Early chess programs also used null move techniques to search more efficiently. Null moves involve evaluating hypothetical move sets as if the player can make two moves in a row. If the player’s position did not improve within two moves, the algorithm removes the first move from its search tree. I thought this quite an interesting way to approach a search and optimisation problem in a game that alternates between two players.
Monte Carlo trees
More modern chess programs today use Monte Carlo tree searches to simulate millions of games and positions. These methods don’t rely on sophisticated rules or knowledge, gravitating instead to positions with a better statistical track record. Monte Carlo search algorithms are popular in games like Go, where permutations are greater and machine evaluation more difficult.
Problems in search
The problem in chess, for both humans and machines, is the vast array of possibilities or ‘branching factor’. So, raw processing speeds and programming efficiency are important factors in a chess program’s strength. The better the hardware and programming got, the broader and deeper the machines could look.
Trade-offs in search
Early computer chess programs faced a challenging tension between effectiveness and efficiency. Computers back then weren’t very fast. Sheer brute force was not yet feasible, making Type B strategies the preferred option. But every permutation it incorporated into its search and optimisation problem increased its processing time. Early chess programs had to choose between “faster and dumber or slower and smarter”.
The classic example is in piece valuation. The simplest of chess programs may value bishop pieces the same as three pawns. While a useful rule of thumb, the guiding policy is not valid in every chess variation. Given the processing constraints, how many conditional statements should we include in our guiding policies to improve our decision-making in general?
Chess is a game of precision. And at the highest of levels, every minor advantage counts. Type B machines could not utilise the guiding policies, intuition, experience, and pattern recognition processes that human chess brains excelled at. But the algorithms of chess programs and processing speeds of computers improved. So, Type-A programs soon overtook Type-B. While chess knowledge and principles were still important, “speed was king”. (For the curious, Kasparov says most modern chess day programs use alpha-beta pruning search algorithms and minimax systems to make decisions.)
Horizon effects
But the brute force strength of Type A programs has its own weaknesses. There are limits to a machine’s search depth due to the vast possibilities of moves. Good players could exploit these chess machines if they planned beyond the machine’s search limit, a “horizon effect”. Hybrid Type A and Type B programs then came about. And smarter search techniques like quiescence search and singular extensions found some success.
Kasparov describes how chess machines excel at sharp tactics, a relative weakness of human players. This is especially so when the configurations are complicated. Computers can process thousands or millions of positions per second. Humans can’t. We just didn’t evolve this way. “Even the human world champion can only see an estimated two or three positions per second.” Our relative strength, as Kasparov sees it, is in planning and positional play.
Alien Grandmasters
Unlike chess machines, humans use strategic thinking and heuristics to make goals, milestones, and plans. Kasparov for examples thinks about ideal positioning or his ‘strategic wish list’ (like moving a bishop to a dominant position or preparing several pieces for an attack). Only then does he evaluate the possibilities and the consequences of getting there.
“Even Grandmasters often fall victim to rote thinking: “Having played A, now I must play B.” A computer doesn’t even know it had just played A; it only ever cares what the strongest move is at the moment. This could be a weakness sometimes, especially in the early phases, which is why they needed opening books. But, overall, their amnesiac’s objectivity made them excellent analysis tools—and dangerous opponents.”
Garry Kasparov, 2017, Deep Thinking.
Kasparov contrasts this to the alien method of machine Grandmasters. Its evaluation function can shift priorities, formations, and strategies at a moment’s notice, regardless of the moves and plans that came beforehand. For machines, this style of objectivity is often an advantage. For a human player with such a style, we might call him or her aimless. Humans haven’t found much success in life playing that way. Kasparov says some planning (and some adaptability) is needed.
“There is a reason we say that a bad plan is better than no plan, at least in human chess. If you have a plan and it fails, you learn something. If you act aimlessly, from move to move, from decision to decision, whether in politics or business or chess, you don’t learn and will never become anything more than a skilful improviser.”
Garry Kasparov, 2017, Deep Thinking
Opening books and table bases
An important element of every Grandmaster’s success is preparation. Based on the styles, strengths, and weaknesses of their opponents and themselves, Grandmasters will develop novelties and innovations to create an advantage. The opening phase in particular combines strategic planning with subtlety and creativity. This is often an area that computers struggle with.
But chess machines circumvent their weaknesses with opening books, a database of human play. As Kasparov describes it, opening books allow computers to ‘skip’ the opening phase and focus on their tactical advantages in the midgame. Opening books provide machines with more options, flexibility and less predictable tendencies. This made machines harder for Grandmasters to prepare against. Humans by contrast have styles, preferences, and tendencies.
Similarly, in certain endgame scenarios, the game of chess is sometimes solvable. There are table bases of solutions that exist today. The seven-piece table bases for example now exist, sitting at a colossal 140 terabytes. Kasparov says some of them may teach chess players and historians something new. But more often than not, the solutions are so complex and convoluted that players would struggle to apply them in practice — another non-issue for powerful computers.
Surviving Deep Blue
While Kasparov found early success in his battles against Deep Blue, the long-term odds were against him. Kasparov, in my view, wasn’t up against just Deep Blue itself. He was facing a team of excellent computer scientists, several chess grandmasters (Deep Blue’s tutors), IBM’s financial backing and the history of chess itself (i.e., opening books and table bases). Deep Blue also benefited from superior computing power, unlimited energy (computers don’t get tired) and silicon-based psychology. It was a formidable opponent then, and even more dominant today with advances in technology.
Following one of his losses to Deep Blue, Kasparov decided to play a “slow, manoeuvring opening”. He didn’t want to give Deep Blue obvious targets or engage in “precision under fire”. These are situations in which the positioning for both players is complex, and the first to make an error is most likely to lose. They involve complicated tactics, which machines excel at.
The greatest art or achievement in chess, the author says, is in “not allowing your opponent to show you what he can do”. Kasparov looked for ways to exploit Deep Blue’s programmed preferences and the horizon effect. He sought “positions in which general principles would outweigh short-term calculations”. But in this specific game, Kasparov’s adaptations meant he had to play a style that wasn’t his. Anti-computer chess was also anti-Kasparov chess.
Gravity of past success
Here’s a great insight and passage from Kasaparov:
“When you have had success, when the status quo favors you, it becomes very hard to voluntarily change your ways… I call this the gravity of past success… Each one of my successes up to that moment was like being dipped in bronze over and over, each success, each layer, making me more rigid and unable to change, and, more importantly, unable to see the need to change.”
Garry Kasparov, 2017, Deep Thinking
Kasparov’s description I think eloquently captures the dangers of success, and how excellence can bring about its own demise. The author recalls, in his championship title loss to Vladimir Kramnik, how he had played into Kramnik’s strengths, refusing to adapt. While Kasparov felt confident going into the match, the reality was that the less-experienced “Kramnik had out prepared [him]”. Similarly, traditional Grandmasters that failed to take advantage of learning and training with a computer found themselves falling behind those that adapted. Whether in chess, business or biology, adaptation is often the difference between survival and failure.
Optimisation and evolution
Here, Kasparov is reminded of Alan Perlis’ Epigrams in Programming, in which he wrote: “optimization hinders evolution”. In programming, optimization focuses on efficiency. It’s unlikely to generate something new. Optimization invokes an opportunity cost. After all, people have to allocate resources between improvement, extension, or adaptation. And in competitive systems like chess or business, excess optimization can mean ruin, leaving one unfit for a changing or unexpected environment. But, as Kasparov wisely notes, Perlis’ adage is only a reminder, not a general rule of life.
Narratives and hindsight
Sometimes it’s difficult to say whether a strategy was good or bad. One problem with narrative analysis, Kasparov says, is our tendency to associate good (bad) moves with good (bad) results. We also like to see the game of chess (and much of life really) as a coherent narrative from start to finish, with a moral at the end.
But this is not always the case. Yes, a loss in chess or poker means that mistakes were made. But the distinction between execution and strategy, your success or an opponent’s failure, is nuanced. Former World Series poker champion Annie Duke described a similar challenge for poker players in her book Thinking in Bets. Both Duke and Kasparov encourage readers to interpret results and narratives with care.
“Everyone loves a good story, even if it flies in the face of objective analysis… It’s important to look beyond the wins and losses. … The moves matter more than the results.”
Garry Kasparov, 2017, Deep Thinking
Human versus machine
The narrative of “human versus machine” is a pervasive one. From the automation of agriculture during the industrial revolution, to the prospect of artificial intelligence in the future, the concern comes in waves. Kasparov’s loss to Deep Blue too raised questions about the future of machines, when just a few decades ago, chess computers were “laughably weak”.
Kasparov describes how “the cycle of automation, fear, and eventual acceptance goes on.” He notes how people were once worried about automatic (operator-less) elevators in the early 1900s. It took quite a bit of time, and a PR push, to change attitudes. The author anticipates a similar trajectory for self-driving vehicles in the near future.
The author also notes that there are plenty of future states between utopian and dystopian predictions of the future. He cites a remark from Bill Gates, who said people tend to “overestimate the change that will occur in the next two years and underestimate the change that will occur in the next ten.”
That’s not to dismiss the legitimate concerns against automation. As Kasparov puts it, “what an observer calls freedom and disruption, a worker calls unemployment”. But we also shouldn’t look to halt the march of technical progress or risk the productivity gains altogether. Kasparov believes the emphasis on transition, education and retraining, as opposed to protectionism and the preservation of the status quo, is the right one.
“The only way to win is to think bigger and to think deeper… We need new frontiers and the will to explore them. Our technology excels at removing the difficulty and uncertainty from our lives, and so we must seek out ever more difficult and uncertain challenges.”
Garry Kasparov, 2017, Deep Thinking
The main line
“If they can get you asking the wrong questions, they don’t have to worry about the answers.”
Pynchon’s Proverbs for Paranoids (Number 3), Gravity’s Rainbow, 1973
Despite all the benefits and wonders of technology, Kasparov wonders whether our reliance on machines might inhibit the way we learn, think and understand? He raises a poignant observation: what do we do when we cannot remember or understand something? Most of us would reach instinctively for our phones.
Kasparov says it helpful to pause and think about our question, “to see if [we] can figure it out on [our] own”. The point is not to know everything ourselves, but to exercise our cognitive muscles. The risk of technology, as he sees it, is in “substituting superficial knowledge for the type of understanding and insight that is required to create new things”.
In the context of chess, Kasparov describes how students are far better players today. This is in part thanks to computers and the internet. But dangers arise when we fail to cultivate understanding through learning and training. Kasparov’s experience with his students portrays it best:
“I will ask one of the students about a move from one of their games, and why he made it. If the move comes early on, the answer is almost always, “Because that’s the main line.” That is, that’s the theoretical move in the database, likely played by many Grandmasters before… But I always ask, why is it the best move? Why did all those Grandmasters play it? … Answering that can take a lot of understanding and a lot of research.”
Garry Kasparov, 2017, Deep Thinking
Because the engine said so…
Given the empirical history and evolution of chess, if Grandmasters have played it, and the computer recommends it, then it’s probably a solid move. But there’s an important distinction between blind application and principled understanding. Without understanding and preparation of that sort, you’ll face grave troubles the moment an opponent introduces a novelty that deviates from your main line.
“It’s not enough to know the best moves; you must also know why those moves are the best”.
Garry Kasparov, 2017, Deep Thinking
As a former economist myself, I couldn’t help but worry when a colleague, client, or consultant made a recommendation because their model said so. Well, why did the model say so? What biases, assumptions and abstractions led to their results? Most do not dare admit that their work and models had degenerated into complex black boxes. I agree with Mervyn King’s view in Radical Uncertainty that the combination of simple models, guiding policies and transparent dialogue can produce more insightful diagnosis and recommendations. Less time doing, and more time thinking can go a long way.
Competition, innovation and edge
Related to the ‘main line’ problem is the issue of innovation and edge. Kasparov illustrates this with a neat example: Let’s say two chess players are replicating the moves of a past game between two Grandmasters. Both players know from history that one player (let’s say black) will eventually lose the game. To win, the black player must introduce an improvement or novelty before the point-of-no-return. But the white player knows this, and may have his or her own countermeasure ready too.
Kasparov says it’s okay for new and younger players “to rest on the shoulders of giants”. But they should also understand that it will not “create anything or innovate in any fundamental way”. After all, it’s easier to imitate than to innovate. And so imitation alone cannot produce superior results at the highest of levels. That’s the nature of competition, learning and adaptation, whether in chess, business, or warfare.
“When it comes to big innovations, you have to start earlier… You have to dig into the tree of established moves that everyone assumes are the best because they’ve been played so many times before… I would also appear with new ideas very early on that occasionally led to a renaissance of a discarded opening or variation. This wasn’t only good for my results, it was good for my feeling of creativity in general, not just in chess.”
Garry Kasparov, 2017, Deep Thinking
Further reading
As you can tell, I really enjoyed Deep Thinking. If you found any of the topics mentioned here interesting, please read or re-read his book in full (especially if you have an interest in chess and business). Short-form posts like these can never capture the richness or joy of reading the original work itself.
To end this post, Kasparov referenced numerous books in Deep Thinking. I highlight the few that I found most interesting:
- Kasparov, Gary. 2007. How Life Imitates Chess.
- Kahneman, Daniel. 2011. Thinking, Fast and Slow.
- Ariely, Dan. 2008. Predictably Irrational.
- Hofstader, Douglas. 1979. Gödel, Escher, Bach: An Eternal Golden Braid.
- Lasker, Emanuel. 1896. Common Sense in Chess.
- Various writings on human and machine cognition from Hans Moravec.
References
- Garry, Kasparov. (2017). Deep Thinking. More books, lectures and events with Garry Kasparov at: < https://www.kasparov.com/ >
You might also enjoy:
- How far do you see? Garry Kasparov on search, calculation, and memory
- Bounded rationality — Herbert Simon on decision-making under uncertainty and complexity
- Only the paranoid survive — Andy Grove on strategic inflection points and super-competitive forces
- The mental models, routines, and checklists of Guy Spier and Aquamarine Capital
- Co-opetition — Brandenburger & Nalebuff on complementors, PARTS and egocentrism
- Arms and Influence — Thomas Schelling on nuclear taboos, salami tactics, and mutual alarm dynamics